Article of the week: External validation of novel magnetic resonance imaging‐based models for prostate cancer prediction
Every week, the Editor-in-Chief selects an Article of the Week from the current issue of BJUI. The abstract is reproduced below and you can click on the button to read the full article, which is freely available to all readers for at least 30 days from the time of this post.
In addition to this post, there is an editorial written by a prominent member of the urological community and a visual abstract created by trainee urologists. Please use the comment buttons below to join the conversation.
If you only have time to read one article this week, we recommend this one.
External validation of novel magnetic resonance imaging‐based models for prostate cancer prediction
Lukas Püllen*, Jan P. Radtke*†, Manuel Wiesenfarth‡, Monique J. Roobol§, Jan F.M. Verbeek§, Axel Wetter¶, Nika Guberina¶, Abhishek Pandey**, Clemens Hüttenbrink**, Stephan Tschirdewahn*, Sascha Pahernik**, Boris A. Hadaschik* and Florian A. Distler**
*Department of Urology, University Hospital Essen, Nordrhein-Westfalen, †Department of Radiology, German Cancer Research Centre (DKFZ), ‡Division of Biostatistics, German Cancer Research Centre (DKFZ), Heidelberg, Germany, §Department of Urology, Erasmus University Medical Centre, Rotterdam, The Netherlands, ¶Department of Radiology, University Hospital Essen, Nordrhein-Westfalen, and **Department of Urology, Paracelsus Medical University, Nuremberg, Nürnberg, Germany
Abstract
Objectives
To validate, in an external cohort, three novel risk models, including the recently updated European Randomized Study of Screening for Prostate Cancer (ERSPC) risk calculator, that combine multiparametric magnetic resonance imaging (mpMRI) and clinical variables to predict clinically significant prostate cancer (PCa).
Patients and Methods
We retrospectively analysed 307 men who underwent mpMRI prior to transperineal ultrasound fusion biopsy between October 2015 and July 2018 at two German centres. mpMRI was rated by Prostate Imaging Reporting and Data System (PI‐RADS) v2.0 and clinically significant PCa was defined as International Society of Urological Pathology Gleason grade group ≥2. The prediction performance of the three models (MRI‐ERSPC‐3/4, and two risk models published by Radtke et al. and Distler et al., ModRad and ModDis) were compared using receiver‐operating characteristic (ROC) curve analyses, with area under the ROC curve (AUC), calibration curve analyses and decision curves used to assess net benefit.
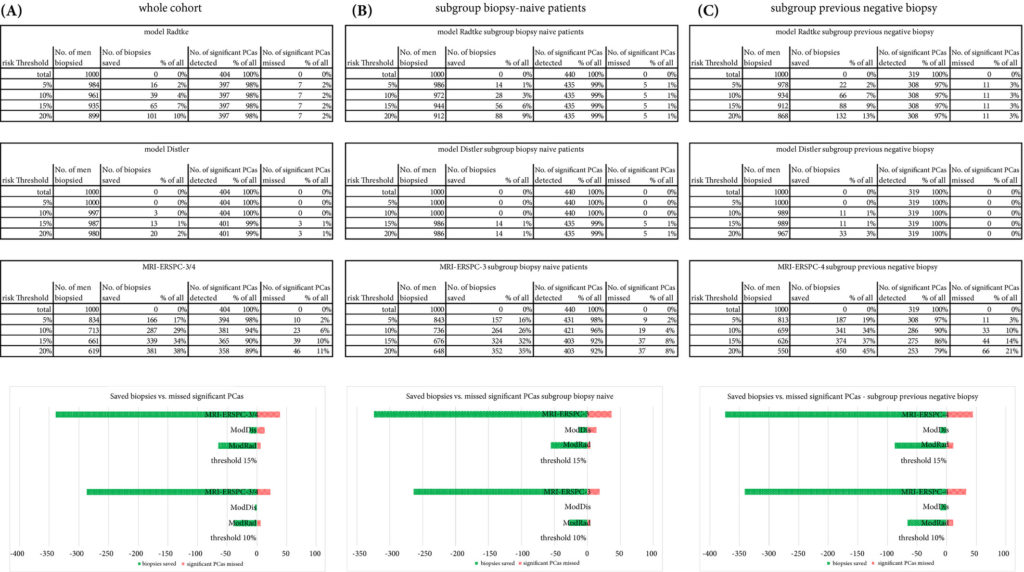
Results
The AUCs of the three novel models (MRI‐ERSPC‐3/4, ModRad and ModDis) were 0.82, 0.85 and 0.83, respectively. Calibration curve analyses showed the best intercept for MRI‐ERSPC‐3 and ‐4 of 0.35 and 0.76. Net benefit analyses indicated clear benefit of the MRI‐ERSPC‐3/4 risk models compared with the other two validated models. The MRI‐ERSPC‐3/4 risk models demonstrated a discrimination benefit for a risk threshold of up to 15% for clinically significant PCa as compared to the other risk models.
Conclusion
In our external validation of three novel prostate cancer risk models, which incorporate mpMRI findings, a head‐to‐head comparison indicated that the MRI‐ERSPC‐3/4 risk model in particular could help to reduce unnecessary biopsies.